Learning-based Model Predictive Control by Exploration and Exploitation in Uncertain Environments
Project funded by the European Union Next-Generation EU (Progetti di Ricerca di Rivelante Interesse Nazionale – Piano Nazionale di Ripresa e Resilienza (PNRR)
The Project
The operation of autonomous systems in uncertain environments is a challenging task. Model Predictive Control (MPC) strategies for uncertain systems have been an active research area in recent years. In the classical approach, given a nominal system model plus some form of uncertainty model, the aim of a robust/stochastic MPC policy is to derive an optimal input sequence, subject to a set of possible evolution scenarios.
In order to improve the knowledge about the system dynamics and the environment or disturbances, learning-based MPC has emerged as a valid alternative to incorporate information into the process model as the control system operates.
Active learning approaches consider in the cost function of the receding horizon problem, both the predicted performance criteria and the future information gain (or loss) about the model uncertainty, caused by the current decisions, leading to a multi-objective problem with an exploitation-exploration trade-off, where the exploitation action aims to optimize performance using the available knowledge about the system, while the exploration effort aims to apply input signals that will produce the most informative outputs to reduce uncertainty.
There are important open questions in the field of learning control systems, e.g., the realization of active learning MPC in nonlinear settings or the derivation of approximated solutions able to be executed in real-time with guaranteed performance and robustness bounds.
The aim of the project is to formulate a framework for the simultaneous learning and control of dynamic systems, able to cope with uncertain and time-variant dynamics or environments, within a receding horizon control framework.
The proposed strategies will integrate active learning actions to exploit online generated information about the process for optimal closed-loop operation, and to avoid the requirement to artificially generate informative conditions and enforced system identification experiments during the operation.
The theoretical guarantees of the resulting control strategy will be verified analytically. Using Set Membership models to bind the uncertainty, the stability, constraints violation risks, and convergence of the learning process will be proved.
The computational cost of the produced algorithms will be studied and adequate simplifications will be adopted to obtain fast execution times for the algorithms, able to be executed on embedded platforms.
Finally, The validity of the developed approaches will be evaluated on two different applications, related to Active noise control and Energy management systems. They will allow the testing of different features of the proposed solutions, both in simulated and experimental environments.
The Team
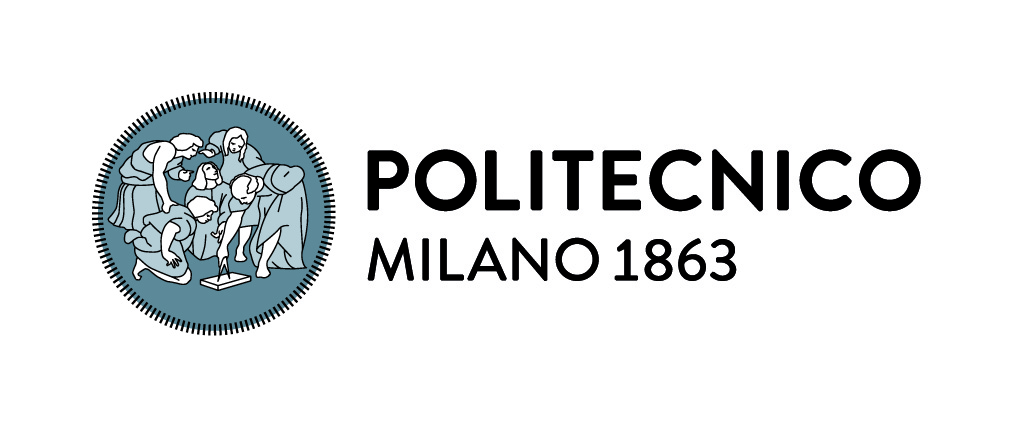
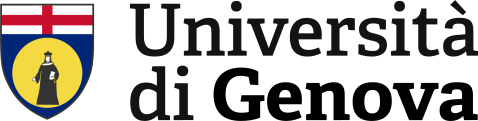
Project funding
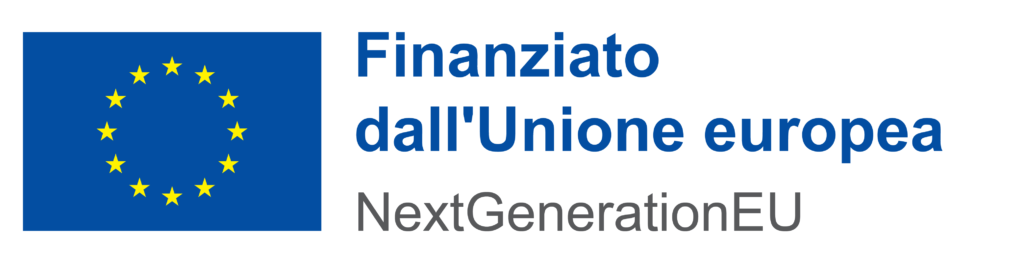
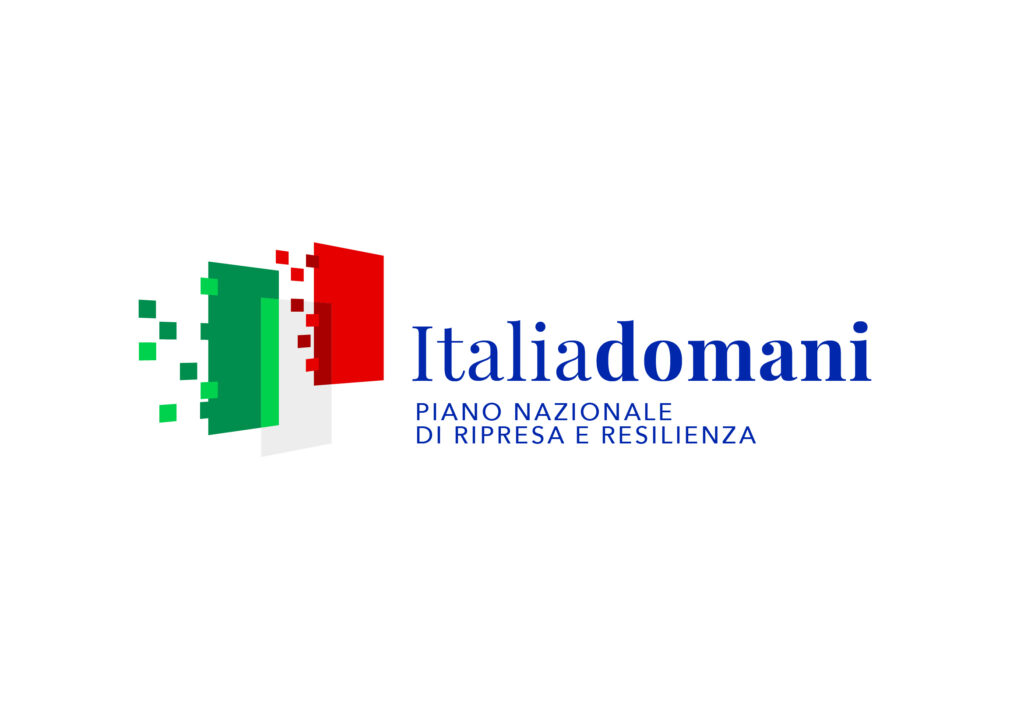
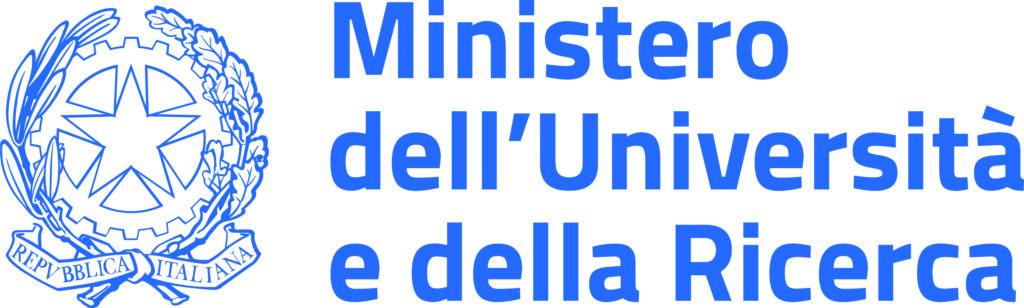